Computational intelligence modeling of hyoscine drug solubility and solvent density in supercritical processing: gradient boosting, extra trees, and random forest models
Por um escritor misterioso
Last updated 22 dezembro 2024
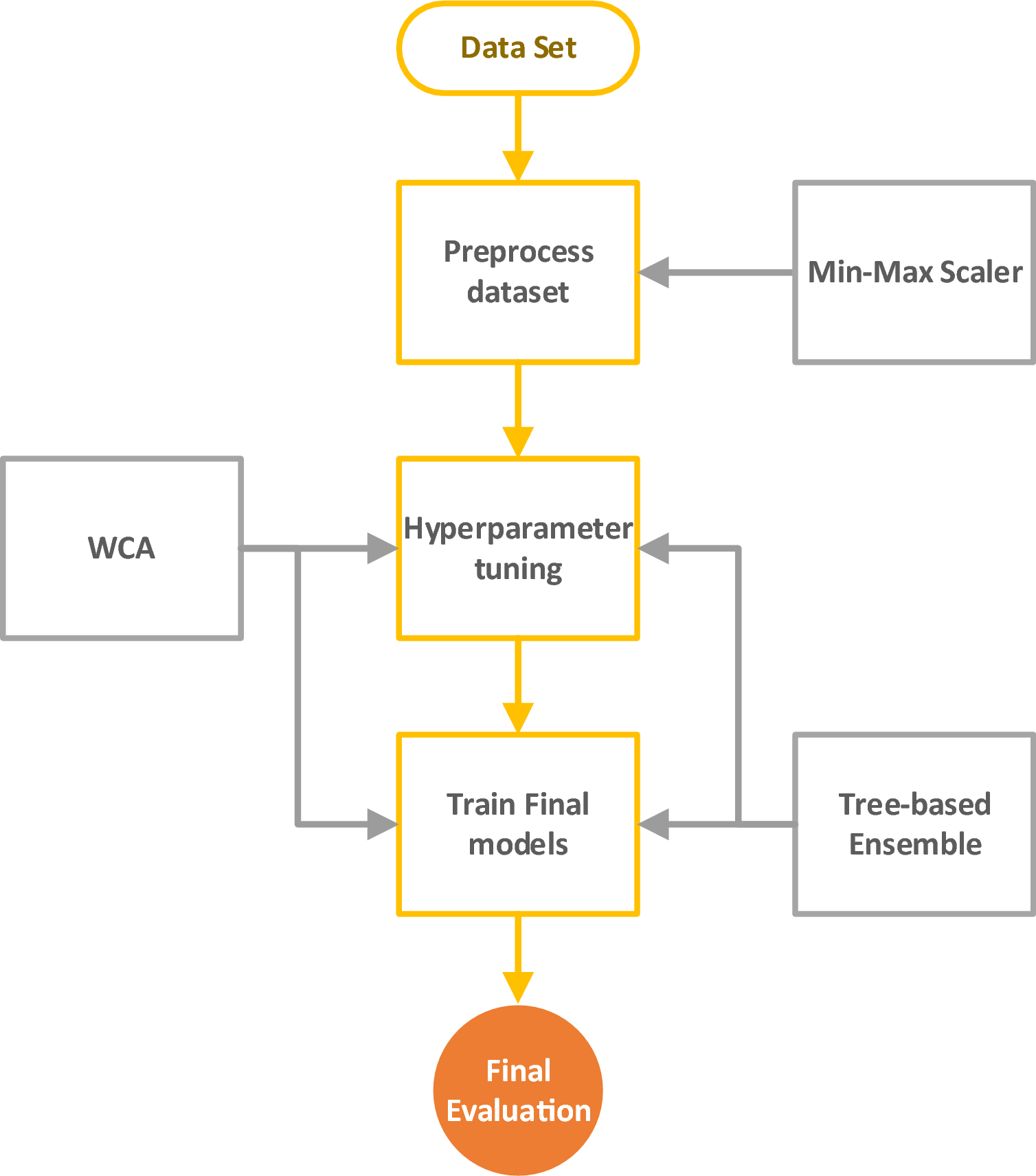

Computational intelligence modeling using Artificial Intelligence

Replacement solvent suggestions for procedures involving benzoic

Data distribution, P (pressure), T (temperature), and Y
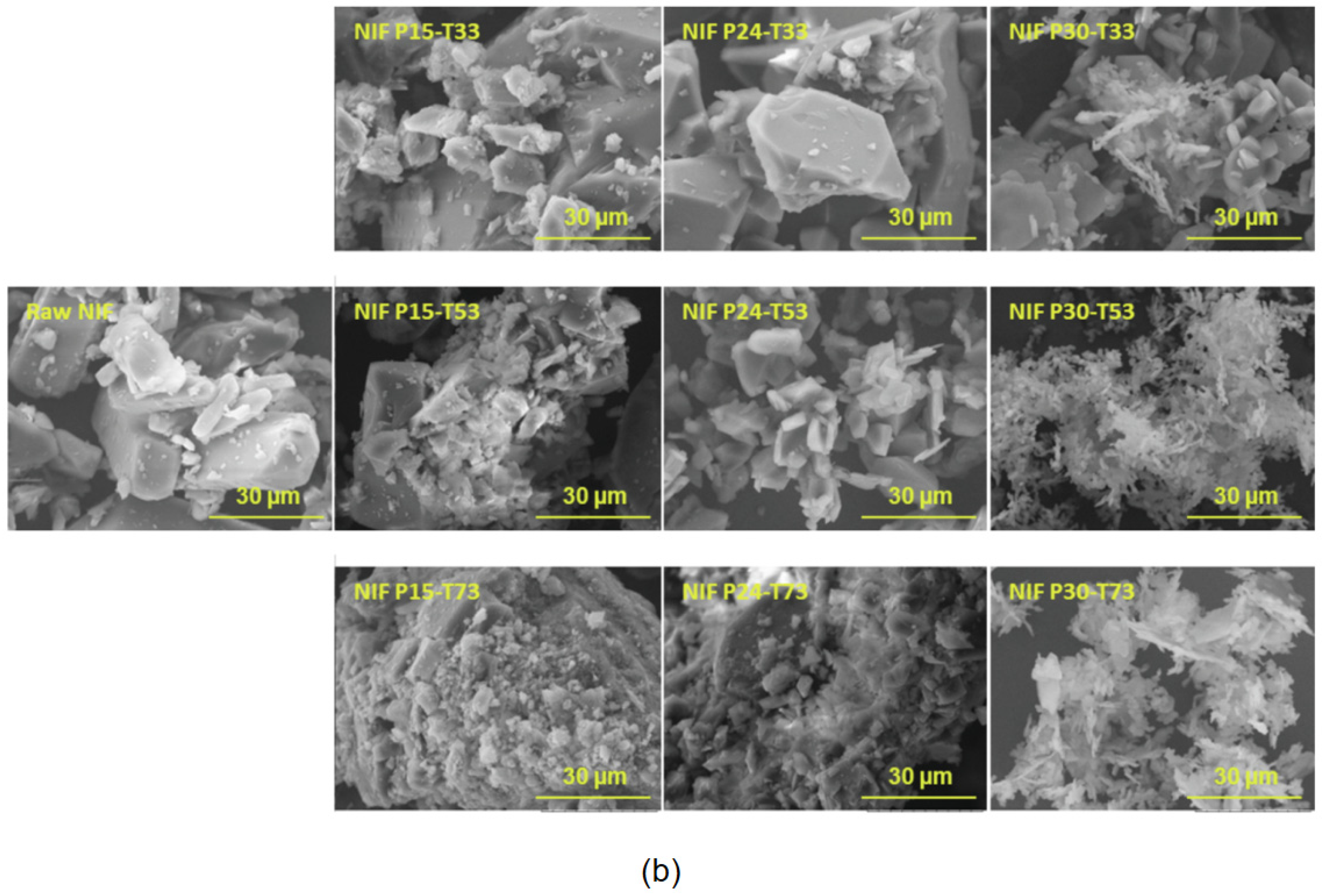
Bioengineering, Free Full-Text

Performance of the models on the existing chemical space of

Representative machine learning algorithms. Machine learning is a

Computational intelligence modeling using Artificial Intelligence
Application of a convolutional neural network (CNN) to classify
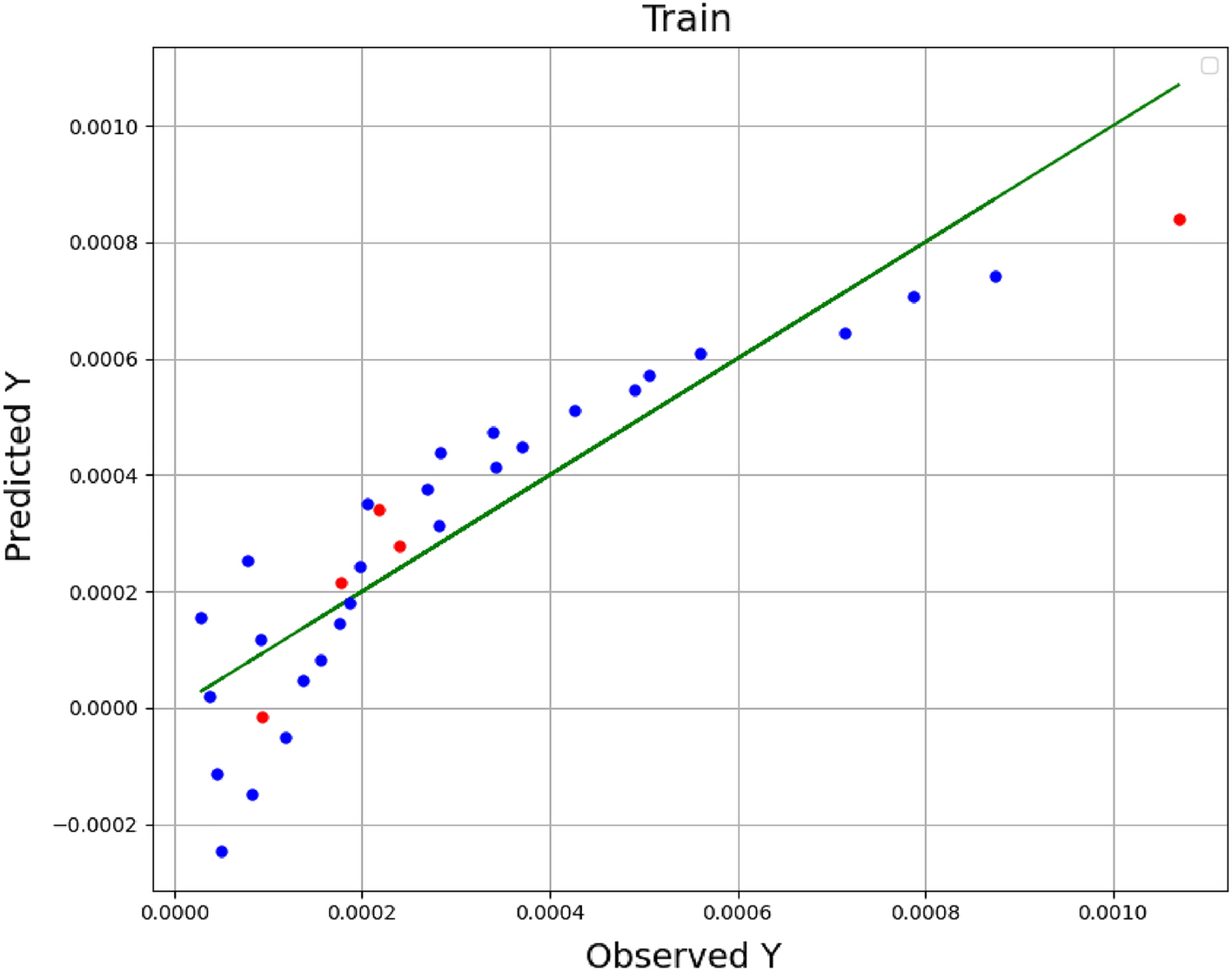
Computational simulation and target prediction studies of

Molecules, Free Full-Text
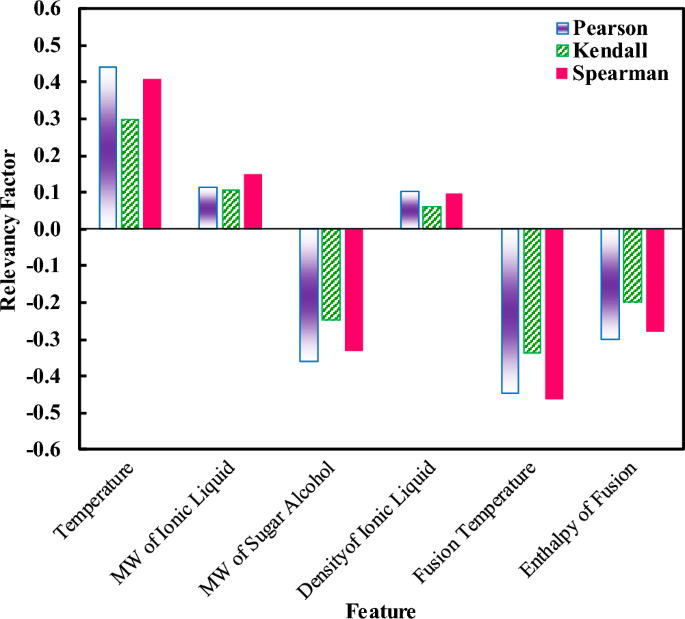
Application of machine learning techniques to the modeling of
Recomendado para você
-
Fort Payne Times-Journal22 dezembro 2024
-
WCA Athletics Williamsburg VA22 dezembro 2024
-
WCA Lions pull away early versus middle school Hornets, Local Sports22 dezembro 2024
-
Ipl Scors - Top, Best University in Jaipur, Rajasthan22 dezembro 2024
-
Connecticut high school football: CIAC Week 8 schedule, stats, rankings, scores & more22 dezembro 2024
-
Demonstration of an Enhanced “Interconnect Topology”-Based Superhydrophobic Surface on 2024 Aluminum Alloy by Femtosecond Laser Ablation and Temperature-Controlled Aging Treatment22 dezembro 2024
-
Chung Y / Tsang W C A TAM C H / NG S Y live score, video stream and H2H results - Sofascore22 dezembro 2024
-
Connecticut high school football: CIAC Week 6 schedule, stats, rankings, scores & more22 dezembro 2024
-
Rewinding Football High Live: Scores, highlights from Week 11 games22 dezembro 2024
-
WCA Conferences Withhold Global Apportionments - United22 dezembro 2024
você pode gostar
-
Big chungus keanu 100 sussy amogus baka : r/EMSmemes22 dezembro 2024
-
Review: Xenoblade Chronicles 3 (Nintendo Switch) – Digitally Downloaded22 dezembro 2024
-
Monster High's G3 Abbey Bominable--A Guest Review!22 dezembro 2024
-
JURASSIC MISSIONS jogos de tiro offline gratuitos versão móvel22 dezembro 2024
-
Pin on PSP games22 dezembro 2024
-
D20-Know No Fear-Warhammer 40K, PDF, Império Romano22 dezembro 2024
-
Barbie makeup cake 💄💖 #barbiecake #tortabarbie #barbiemakeupcake22 dezembro 2024
-
Hatsune Miku gacha life 2 by YourLocalArtist88 on DeviantArt22 dezembro 2024
-
Trecho do modelo de tradução inglês-português aprendido a partir22 dezembro 2024
-
Fonte Gamemax 600W, 80 Plus Bronze, Semi-Modular - GM600 P-1629 - Fonte Gamemax 600W, 80 Plus Bronze, Semi-Modular - GM600 - Gamemax22 dezembro 2024